Artificial Intelligence applied to railway fleets: Reduce LCC and optimize vehicle operation
AI is one of the keys to achieve a successful reduction of trains LCC (Life Cycle Cost) and to optimize vehicle operation by increasing availability and reducing incidents in service. We say ‘key’ and not ‘ultimate’ because AI does not work alone. It is not a force that takes over and gives maintainers and operators the instructions to achieve their goals, but a data-based tool that works in combination with other powerful tools to help increase availability, reduce service incidents, and provide a higher quality service, reaching the two main goals when using AI in the railway industry: reducing the LCC of the train and optimizing the operation of the vehicle.
Our solutions are mainly aimed at facilitating correct decision-making by the agents involved, providing relevant insights that allow them to make the best possible decision of the current situational context of their fleet. Bearing in mind the vast amount of information collected from a fleet and other systems, exploiting all of this would be an impossible job without the help of AI, which makes sense of it. This makes Artificial Intelligence one of the major factors in being able to use all data to improve overall functions of the service.
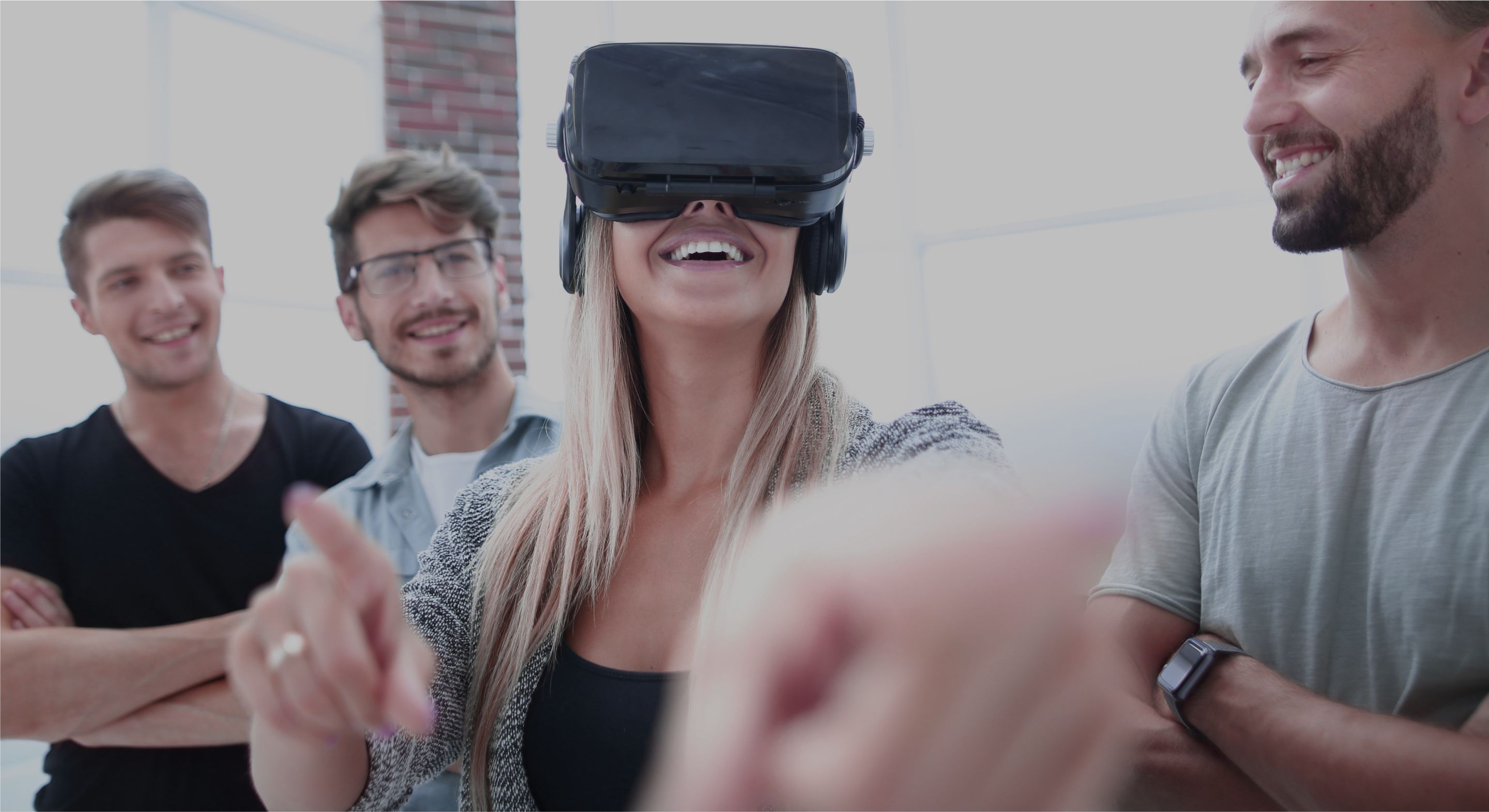
What does this mean for maintainers and operators?
Maintainers obtain a significant advantage as they are able to identify incipient symptoms of failures, thanks to already deployed products, before they become service incidents or more serious breakdowns. This is possible thanks to the exploitation of all relevant data gathered from the train and the deployment of AI models able to identify anomalies in the data coming from the sensors. However, these models must be integrated with existing workshop processes in order to capture all their value. In this regard, automatic generation of work orders triggered by alerts coming from these models along with a process in place able to handle the inherent uncertainty associated with these tools are key points. Additionally, these models are being improved (and validated) thanks to the feedback of the workshops which is being gathered thanks to the processes in place.
Some of the current Artificial Intelligence models implemented in this regard are:
- Detection of anomalies in train systems with the information collected on board the train to identify equipment that may have an incipient failure. This helps the workshop to schedule better the maintenance operations and avoid unnecessary downtimes.
- Wheel life prediction to optimize the remaining life of the asset and schedule more easily all the maintenance activities related with this asset.
- Detection of defects in railway components using artificial vision in order to reduce manual inspections and increase safety thanks to a regular checking on almost a daily basis.
- Optimal task planning, assisting operators to take into account the various sources of information (drivers, preventive maintenance plan, analytics-based warnings...), reduce maintenance operations and increase availability.
- Optimizing energy consumption. In this regard, knowing the energy consumption of each train, in each operational state and identifying in each situation the consumption of the different systems of the train helps them understand where there is potential for energy and cost savings. Furthermore, minimizing energy consumption is possible with the help of a train digital twin to model energy consumption. This digital twin enables the operator to understand how the train really behaves and compute the optimal way to operate the train subject to the operational constraints to reduce energy consumption.
In conclusion: CAF’s LeadMind solutions are oriented towards helping decision-makers, maintainers, and operators, to ensure that the right action is taken in each and every situation to achieve their purpose. All of this with an efficient use of resources.
AI in rail projects: Know about real cases
With all these improvements and works as an ambition, and for some time now, within CAF there have been a series of projects that serve as spearheads to develop and implement this type of solutions and evaluate their impact on the business. From tram fleets such as the one in Zaragoza, metro fleets such as Santiago de Chile or medium distance such as in Euskotren, in Sao Paulo or in some of CAF's recent UK projects. LeadMind has been deployed in 80% of CAF’s maintenance contracts
As said previously, these tools aim at reducing the LCC cost of the train. Alfonso Moreno, Lead Data Scientist at CAF DS, mentions “one of the main focus to achieve this is via identifying the condition of the systems of the train to reduce incidents in service, avoid unnecessary maintenance inspections and reduce the work associated with regular maintenance tasks. In this regard, there are models in place to identify anomalies in the telemetry and HMI alarms generated by the equipment that helps to spot points of interest the workshop probably should pay attention to”. He adds: "for example, in a project in Sao Paulo an AI model has been deployed by CAF’s data scientists to allow the operators and maintainers better exploit and make better use of the vast amount of information the trains HMI yields".
+imagen
This product alerts of suspicious maintenance alarm activation patterns and gives the maintainer a more accurate view of which systems in the fleet are likely to require attention either because a real problem exists that is in need of attention or because there is an anomaly or malfunction with the alarm.
According to Alfonso, ‘given the good feedback from the business, this product has been deployed in other projects where CAF is responsible for maintenance, and although very incipient, the results seem to be along the same lines.’
The models to identify incipient failures in the HVAC are another good example. Different AI models have been deployed and can detect anomalies on the air conditioning system with an accuracy over the 80%, which makes it possible to see incipient faults in this equipment many times before the passengers themselves perceive anything from the point of view of their comfort.
Moreover, these models do not simply show when an equipment may have a problem but provide an explanation as to why they consider the behaviour of the equipment to be abnormal. This allows the maintainer to have a clearer idea of what the fault may be, managing both the equipment spare parts logistics and the appropriate personnel in charge of reparations and maintenance in a timely manner.
Regarding this, Moreno adds: ‘these result in less time to resolve the malfunction and in a clear reduction in the number of repeated actions on the same equipment due to an incorrect repair. We have similar solutions deployed on other equipment such as powerpacks on diesel train fleets in the UK. And we are in the process of developing them for the rest of the train systems.’
A glimpse into AI's near future (and present)
Artificial Vision
In the field of operational efficiency, CAF is developing an ambitious artificial vision project. In due time, artificial vision is expected to reduce significantly the number of manual visual inspections required, which will enable a significant increase in the availability of the vehicles. Moreover, this system, once it is in place, will also result in safety increases.
Human in the loop
Feedback is the core of AI. Feedback provided by humans on every operation is what allows AI to learn and improve, so that better inputs for decision making can be provided. In the data world, this is known as ‘Human in the Loop’ and it is the easiest way to ensure that these models improve and scale. Alfonso Moreno highlights, 'a scalable way to gather when the outputs of these models are good and when they are not is key to make big steps forward with regard to the capability of prediction of these models.'
On the other hand, it is important to mix well how the end users can exploit the outputs of these models. In the end, it is important to keep in mind that the final decision does not rest with an AI, but with a real person, either on the spot, with the help of the information provided by the AI, as for example in the case of deciding how critical an anomaly detected in a piece of equipment is in order to schedule its repair, or because it is pre-configured.
Predictive maintenance models and digital twins
A digital twin is a digital representation of a real system using data provided from it, and it falls into the category of predictive maintenance models that are being continuously deployed and developed in CAF. The intention of a digital twin is to evaluate the state of a piece of equipment in order to anticipate the need for any kind of maintenance.
CAF is moving forward with projects that fall under the digital twin category: one to optimize the maintenance of the railway wheel and another to minimize the train's energy consumption.
Today, CAF has developed models that optimally predict when to perform certain maintenance works on the wheel to fulfil the contract, and these same models are able to detect if a wheel is not reaching its life expectancy so corrective measures can be applied early. Data plays an important role in this process and positive results have led to major cost savings.
Wheels are fundamental assets of fleets. It is also a great example of how data contributes to solving multifaceted problems. The railway wheel is affected by many circumstances and not all of them are in the hands of the manufacturer (the track, the weather, the operation, the maintenance plan...), but in the end, the manufacturer is the one responsible for designing, manufacturing, and ensuring a wheel with an adequate duration and performance.
Regarding the digital energy twin, this uses physical models that are fed with real data from the operation of the train. This model allows to obtain the optimal train speed profile from an energy point of view, subject to the fact that the train has to comply with a schedule and that certain traffic limitations must be met. Preliminary data suggest potential savings of more than 10% thanks to this type of optimization.
This digital twin is built upon both knowledge of vehicle design and the information provided by train telemetry and alarms, which, with the help of ML models, enable the operator to understand the train needs for energy.